Educated decisions are informed by data, which is often originated or stored in spreadsheets. Even small discrepancies might, over time, lead to high deviation from the reality reinforced by misinformed actions.
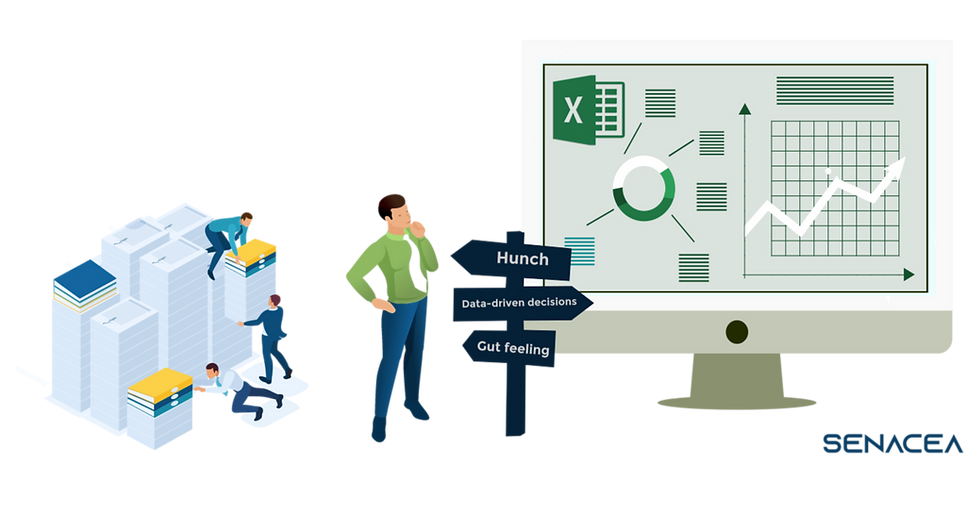
Decision-makers rarely process data in Excel
In many companies, one person would transform raw data into insights, and others would authorise actions based on it. Therefore the first of them has the duty to accurately present the comprehensive analytical picture going beyond just a plain dashboard or spreadsheet full of tables. Providing the full context and explanation of the methodology used is crucial for avoiding the loss of relevant information.
Data origination, processing & visualisation determine decision quality
Even the best strategists will make bad decisions when misinformed. Also, in the perfect world, those that act on data insights would not have to audit or investigate them. Following these 10 best practices will make your data insights actionable and useful for decision making.
1. Data presentation matching the audience
Choose the appropriate level of detail and visual representations. If your audience is a knowledgable executive, prepare high-level reports that allow investigating the issues of their interest. If you are providing insights about the use of your services to a B2B customer, include only relevant data that won't be cumbersome to interpret. Be actionable and precise, also when it comes to descriptions.
2. Top to bottom access
Spreadsheets allowing users to see the overview of insights and then to investigate those aspects that interest them or don't match others. Top to bottom approach is a good compromise between informational overload and insufficient granularity of insights.
3. Visual combination aiding decision making
It's commonly said that the human brain may store only up to seven pieces of information at any one time. It is hard to draw conclusions based on data scattered across multiple worksheets. The right presentation of insights requires pulling together information that is analysed jointly.
4. Eliminating data noise
Using different templates or originating data on a big scale makes it easy to calculate a multitude of indicators and ratios. The challenge is to present only those relevant and give optional access to those less important if necessary. That also involves focusing on the components most actionable and highest in value. You wouldn't dedicate 30% of your spreadsheet report to sales category, making up 1% of revenues that the company has no influence over.
5. Facilitating apple to apple comparisons
As businesses pivot and leaders change, the business landscape also changes. When projecting future sales or comparing next year budget with previous years, it is not easy to compare those values properly. The right spreadsheet structure clearly addresses those differences visually and with concise descriptions. It might mean mentioning a new breakdown of sales regions or one business units overtaking certain processes.
6. Clear classification, data description & naming methodology
Always properly document naming conventions and the criteria for data classification. Otherwise, likely, somebody in the future will not understand something as clearly as you. Is the growth in per cents or percentage points? What does that 3-letter acronym mean? What are the exact thresholds for the purchase to fall into the "large sum expenses" category?
7. The appropriate level of detail
When analysing time series, choose the right scale. For the overview of 3-year cashflows, you might choose monthly intervals. Customer service KPIs might require a daily breakdown. Merge smaller categories and break down the major ones. Remember that an inaccurate level of granularity and detail might distort the interpretation of data.
8. Including event-related and qualitative data
Quantitative data has to be supported by qualitative data. Whether it is a model with a binary or Likert scale variable or just volume analysis, explain all the anomalies. Maybe a sudden increase in sales of a certain product is the result of a single review from a popular trendsetter? Or maybe the competitor ran out of their supplies and our product replaced theirs for several weeks? Exception handling also prevents distortion of the model where incorrect attribution might inflate regression coefficients.
9. Feedback loops embedded in all data handling steps
At a minimum, data is originated, processed and then interpreted. Very often, by three different professionals or parties. Coordinating this process and tackling inefficiencies at the source is often much better than constantly repairing data errors. When you receive data feed spreadsheets that require extensive cleaning, you might want to constraint the inputs gathered by data collection teams. If drawing conclusions from dashboards takes much time, a conversation with analyst might fix that.
10. Leveraging data, not indulging in it
This one is more about philosophy. Some analysts like their data too much and want to utilise it or never change the ways they process it. And data is not something static and not the goal itself. The collection and processing methods may be and should be changed with the right business justification. Don't get attached to your data. Instead of curating it, cleanse, leverage and interrogate it. And don't be afraid of abandoning or not using some data sets if they do not provide any relevant value.
Epilogue
It takes time and effort to develop high standards of data processing to inform decisions optimally. Going beyond siloed structures where different teams work on data at different stages of its processing is increasingly difficult. If you don't have specialists that coordinate the entire route of data, consider hiring Excel Experts. If you would like to learn more about how to improve spreadsheet use in your company, read our comprehensive guide to efficient Excel.
Comments